Wharton Sports Research Journal
2025 Spring Edition
The papers in this issue include research from students at the University of Pennsylvania as well as high schools and universities across the country, ranging across sports and statistical techniques, including softball and Paralympic sports.
Analyze Tennis Winning Factors Across Different Surfaces By Utilizing Random Forest
Author: Wuhuan Deng
Department of Applied Mathematics, University of Washington ’25
To what extent do socioeconomic factors such as HDI, continental origin, and previous host advantage affect paralympic medal winning?
Authors:
Ahmed Sherif Elagamawy, International Programs School ‘27
Valerie Caniedo Mangao, International Programs School ‘26
Muhammad Haider Shabaz, International Programs School ‘26
Defensive Motor Index (DMI): A New Metric for Evaluating Defensive Effort and Impact in the NBA
Author: Pratik Gurijala
Liberal Arts and Science Academy ’27
Using Machine Learning to Construct Optimal Team Rosters in the Modern NBA
Author: Jaden Patel
St. Paul’s School ’26
Testing the ‘Bottleneck’ Hypothesis in Professional Tennis Rankings
Authors:
Seth Richey, Florida State University ’25
Ryan Rodenberg, Florida State University
Stats & Stumps: Using Machine Learning to Predict T20I Matches with Player and Venue Data
Author: Archith Sharma
Texas Academy of Mathematics and Science ’25
Adjusting Double Poisson Models to Predict the NCAA Division I Softball Championship
Authors:
Liam Smith, The University of Alabama ’26, Randall Research Scholars Program
Brendan Ames, University of Southampton, School of Mathematical Sciences
The Risk and the Reward: An In-depth Evaluation of Strategic Aggressiveness in Men’s Tennis
Authors:
Atul Venkatesh, Dartmouth College ’26
Aahan Mehra, Tufts University ’27
Deal or No Deal? – How NFL Teams May Be Better Off in the Draft
Author: Shreyas Vinchurkar
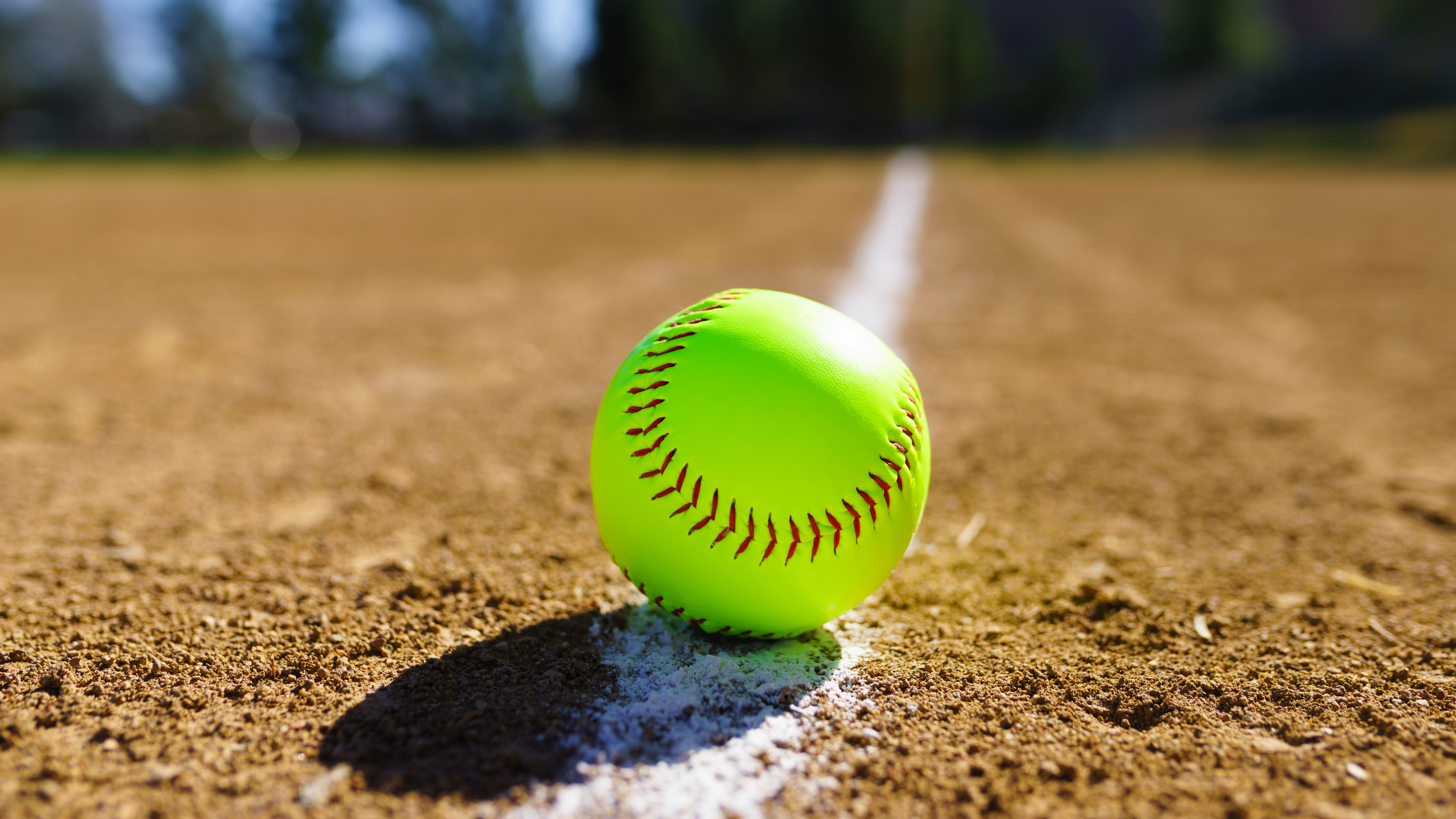
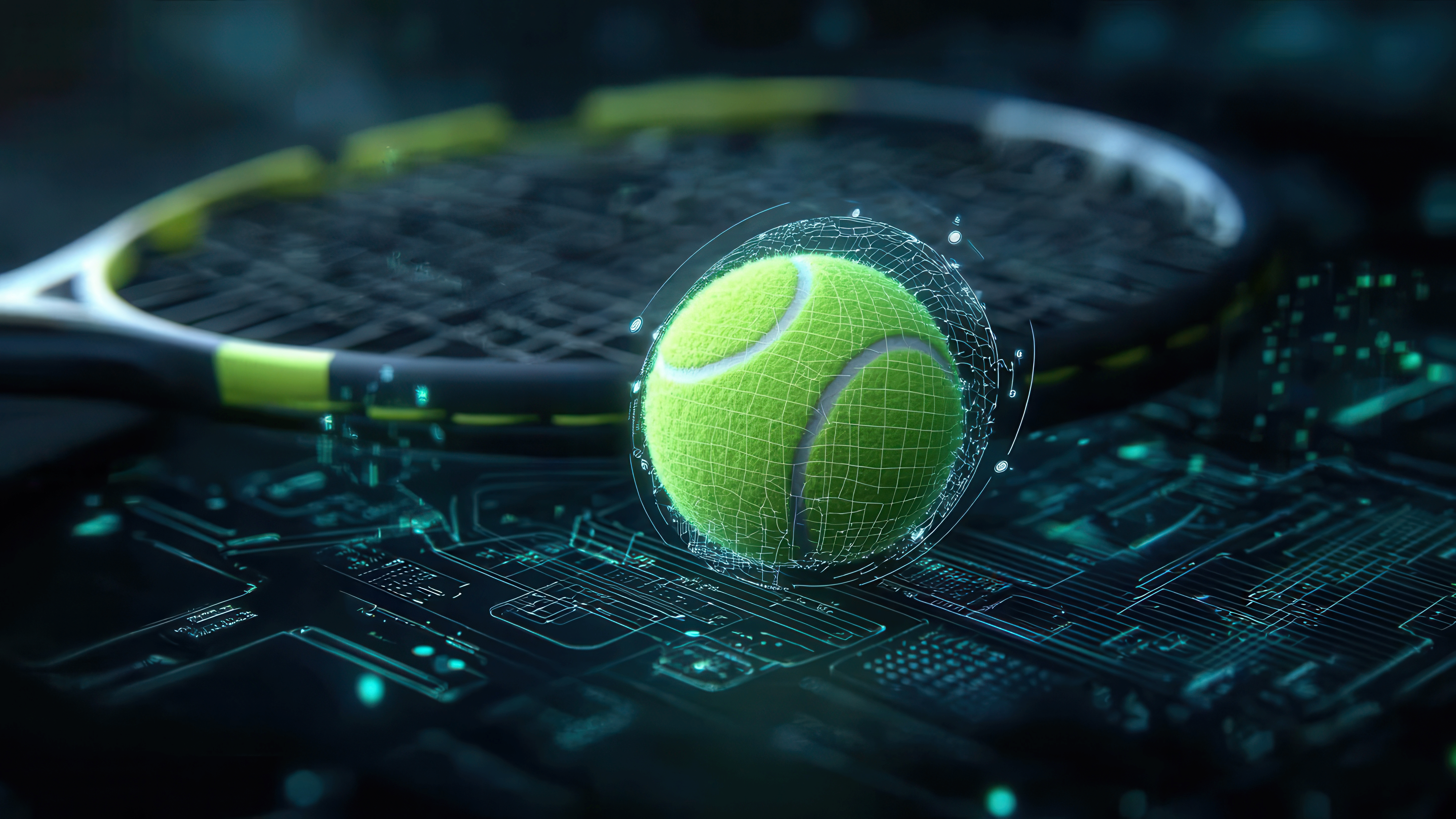
Analyze Tennis Winning Factors Across Different Surfaces By Utilizing Random Forest
Wuhuan Deng
Tennis is one of the most popular sports worldwide, with a rich calendar of professional tournaments played across three court surfaces: hard, grass, and clay. Each surface has unique physical characteristics that significantly influence ball behavior, player movement, and match dynamics. As a result, different playing styles tend to be more effective on certain surfaces.
This research investigates the surface-dependent nature of match outcomes by exploring statistical trends and performance indicators that contribute to success on each court type. Understanding these differences can provide deeper insights into player adaptability, match strategies, and surface-specific training.
To what extent do socioeconomic factors such as HDI, continental origin, and previous host advantage affect paralympic medal winning?
Ahmed Sherif Elagamawy, Valerie Caniedo Mangao, Muhammad Haider Shabaz
This research examines the extent to which socioeconomic factors – HDI (Human Development Index), continental origin, and previous host status – affect medal distribution. This study takes the most recent 2024 Paris Paralympics as the focus, with the tests being conducted on secondary data collected from the official Paralympics website. A multitude of statistical methods and analyses were utilised to test the data, such as the Theil Index, standard deviation, etc.
The research identifies significant disparities and deviations in the Paralympic medal dispersion. The findings of this study reveal that nations with higher HDI scores, primarily those in Europe and North America, win a significant large proportion of the medal count, while countries with lower HDI scores originating from other continents (Africa, South America, Asia) tend to struggle and face significant hurdles.
Defensive Motor Index (DMI): A New Metric for Evaluating Defensive Effort and Impact in the NBA
Pratik Gurijala
Defense in basketball is often difficult to quantify due to its reliance on effort, positioning, and hustle plays that do not always appear in traditional stat sheets. This paper introduces a novel metric, Defensive Motor Index (DMI), designed to evaluate a player’s defensive effort and impact beyond basic statistics. DMI integrates hustle statistics such as deflections, loose ball recoveries, contested shots, and defensive transition effectiveness. By applying DMI to NBA player data, this study highlights undervalued defensive contributors and provides teams with a better tool for assessing defensive performance.
Using Machine Learning to Construct Optimal Team Rosters in the Modern NBA
Jaden Patel
This study analyzes NBA roster composition over a 10-year period (2014/15 to 2023/24), aiming to identify optimal player archetypes and positional balances for maximizing team success. Detailed individual and collective performance and physical trait data from 300 distinct teams and 3557 players was used. Players were clustered into ten archetypes and three general positions (Guards, Wings, and Bigs) through k-means clustering. A supervised learning (gradient boosting) model was then employed to predict team win totals based on archetype and position profiles.
Results highlight the critical role of 3-point Specialists and Defensive Wings in modern NBA success, underscoring the value of versatile, low cost players – role players who contribute on both ends of the floor.
Testing the ‘Bottleneck’ Hypothesis in Professional Tennis Rankings
Seth Richey and Ryan Rodenberg
We test whether recent policy changes by the governing body of men’s professional tennis—the ATP Tour—have created a statistical incongruence in the ordinal ranking of players worldwide. Using a quartet of parsimonious methods, we find prima facie evidence of a so-called ‘bottleneck’ in the ATP Tour men’s singles rankings consistent with publicly acknowledged criticism of the player evaluation system following alteration of the ranking point distribution schedule between the 2023 season and the 2024 season.
Specifically, we pinpoint that #100 in the men’s singles rankings exhibits characteristics consistent with a bottleneck that would seemingly impact meritorious promotion and relegation within the sport. Our findings highlight the importance of using sports analytics when designing sport governance models and evaluating the impact of major policy revisions.
Stats & Stumps: Using Machine Learning to Predict T20I Matches 1 with Player and Venue Data
Archith Sharma
Cricket is gaining popularity worldwide rapidly, and at the front is the newest format of the game, Twenty20 Internationals (T20I), and big data. This project attempts to predict cricket match outcomes using player-level performance metrics and machine learning models.
A dataset of 1,029 T20I matches was analyzed, with player-level features engineered from batting and bowling statistics such as runs, strike rate, boundaries, wickets, economy rate, and maiden overs.
Adjusting Double Poisson Models to Predict the NCAA Division I Softball Championship
Liam Smith and Brendan Ames
While its viewership has surged in recent years, college softball remains an under-researched sport in the domain of sport analytics, partially due to a lack of a longstanding major professional league. However, the postseason format of major college softball – a four-stage layout with two four-team double elimination phases and two best-of-three series – presents an intriguing challenge for predictive models.
Primarily focusing on the first of these four stages, we evaluate the effectiveness of a Double Poisson model in predicting the outcome of this competition.
The Risk and the Reward: An In-depth Evaluation of Strategic Aggressiveness in Men’s Tennis
Atul Venkatesh and Aahan Mehra
In a tennis match, when do players know the right situation to be aggressive? The role of aggressiveness on in-game tennis strategy is one of the most overlooked aspects of the sport.
Using shot-by-shot data, we seek to answer the following question: holding ranking constant, based on the in-game situation, what level of aggressiveness is most strategic and yields the largest reward?
Deal or No Deal? - How NFL Teams May Be Better Off in the Draft
Shreyas Vinchurkar
In this research paper, we will identify the best strategies that successful teams use, to win playoff games and make profits for the team. It aims to uncover how strategic decision-making in team management influences both on-field performance and financial outcomes, particularly in relation to market size and economic factors .
Through the use of historical data from the past decade, trends in draft picks, free agency spending, and team performance metrics were uncovered. Case studies of marquee draft selections and their immediate economic effects on teams were also included. Additionally, economic variables such as market size, gross domestic product(GDP), and disposable income in metropolitan areas were examined to evaluate their influence on revenue patterns.
Our findings demonstrate that larger markets, such as New York and Los Angeles, maintain consistently high revenue irrespective of team performance, whereas smaller markets exhibit a strong correlation between revenue and on-field success.